Researchers have shown how ‘network alignment’ techniques can be used to map nodes from a reference graph into an anonymized social-network graph. These algorithms, however, are often sensitive to larger network sizes, the number of seeds, and noise~— which may be added to preserve privacy. We propose a divide-and-conquer approach to strengthen the power of such algorithms. Our approach partitions the networks into ‘communities’ and performs a two-stage mapping: first at the community level, and then for the entire network. Through extensive simulation on real-world social network datasets, we show how such community-aware network alignment improves de-anonymization performance under high levels of noise, large network sizes, and a low number of seeds. Read more in our paper, which will be presented at ACM CCS 2014.
Our Work on Community-Enhanced Deanonymization to Appear at CCS 2014
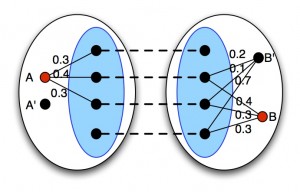